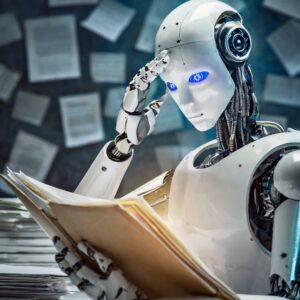
What AI should be doing with documents
It’s time for AI integrators to help authors make semantically rich documents… and step up their game on PDF inputs.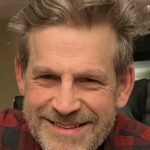
“Best-practice PDF is also the best PDF for AI.”
In the 1990s, optical character recognition (OCR) got a lot of attention, enabling search engines to work on scanned documents, providing near-instant access to relevant content without laborious manual indexing.
In the mid-to-late 1990s, I owned an imaging service bureau. We delivered OCR output in addition to scanning services. In selling these services it was very important to be able to help customers understand vendors’ accuracy statistics.
OCR’s power was real, but it wasn’t a panacea. Recognition errors led to misses or false positives. These problems were eventually alleviated, in part, by additional software such as language identification and dictionary lookups that post-processed OCR results to improve accuracy. But one fundamental problem was (and remains) harder to solve - complacency. As academic research on the subject has demonstrated, the magic of OCR and search engines inspired far more trust in these systems than the results warranted, leading in some cases to costly mistakes.
Trust in extraordinary technology should not be blindly given, but a key lesson of the information age is that the easier the technology is to use the more readily it gets trusted, even if that trust isn’t earned.
AI’s promise is great, its risks are likewise great
Although Artificial Intelligence (AI) is beginning to dramatically transform how users interact with and process documents, weaknesses remain. Even when the training data is tightly quality controlled, AI’s results are not necessarily trustworthy.
Although AI is already assisting in authoring, data extraction, and content management, AI tools can only be as good as their inputs and training. If input data is biased, AI models are helpless to resolve - or even detect - the problem, a major source of AI hallucinations. But, as Air Canada, Michael Cohen, and many others have already realized, relying on AI for critical tasks should prompt far more due diligence than their ease of use implies. AI is a near-miraculous assistant, but trust must be earned, not given.
Competent processing of inputs is a sine qua non for competent, trustworthy AI. So far, however, we see AI developers relying on data volume and (apparently) ignoring data quality.
It’s not the AI’s fault - it’s what they are fed
While the vast majority of content available for training might be unstructured or poorly semantically structured, this doesn’t mean that the semantic information it includes should be ignored. When proper semantics are present in a document, such as with WAI-ARIA or Tagged PDF, this information becomes a far richer source of trusted knowledge for AI, whereas attempting to retrospectively guess can result in the familiar garbage-in-garbage-out (GIGO) problem.
If AI assistants helped creators to make richly structured documents - and if consuming AIs were equipped to leverage such enriched inputs, including associated machine-readable source data and provenance information, results would evolve to become more trustworthy.
However, document authoring tends to be oriented towards visual consumption, with unstructured or unreliably structured content. Yes, today’s AI assistants can help recognize headings and paragraphs and apply these simple semantics for novice users, but the richer semantics of quotes, referencing, indexing, math, illustrations, etc. do not get the same level of attention, Even structured source information for tabular data (say, an Excel file) is commonly published in unstructured ways while the associated source data is rarely published at all.
This problem is less acute with HTML, as semantic structures are commonly integrated into the content, offloading the complexities of determining appearance to the browser. PDF is necessarily a more complex format than HTML because it’s self-contained. That’s why good-quality PDF files and a full PDF parser is essential to extracting anything useful from PDF.
The choice of parser matters… a lot. There are literally thousands of PDF parsers covering a vast array of applications and cases…. but only a relative few are truly competent at ingesting PDF content in all its variety. If your parser doesn’t support all versions of PDF, uses old Unicode or outdated CMaps, can’t understand Tagged PDF, ignores annotations, doesn’t process language markers, has no idea about right-to-left or vertically typeset languages, … then inputs into AI will be biased accordingly.
How should AI be integrated for use with documents?
For creators
AI integration should focus on helping content creators to not only draft and refine their content but also to richly structure and contextualize it. Beyond recognizing tables and lists and offering to structure them, authoring applications should:
- identify quotations, and include the source (either visibly or as metadata);
- when pasting content, include the source (either visibly or as metadata);
- recognize math, and include MathML, even when equation editors are not used;
- expose the AI’s confidence in AI-generated alt text of images, and ask the author to review;
- recognize abbreviations and acronyms (does “Dr.” mean Doctor or Drive)?
- suggest improvements to the document’s structure and ensure appropriate document navigation;
- ensure metadata, referencing and cross-referencing all remain meaningful to the content as it is edited;
- recognize the intentions behind character formatting (are bold words emphasis or defined terms, etc.) and embed those semantics;
- ensure hyphenation and whitespace is semantically indicated;
- retain the semantics of common tools such as org-charts, flow-charts, and drawing tools (these are not just lines and words, but represent semantics!)
- in slide decks and document templates, ensure that page “chrome” is semantically identified;
- ensure that generated PDF files include all this information via Tagged PDF, embedded and associated files, links to structured sources, document and object metadata, ARIA roles and C2PA and digital signatures for provenance and authentication.
At the bare minimum, born-digital documents created using AI assistants, regardless of format, should include the full range of accessibility features such as meaningful alternate text for images, logically arranged headings, and MathML for mathematical equations, to name a few. They should also provide open data to support any graphs and charts. We already see some of these features today in various modern office suites, but the author-side AIs aren’t (yet) necessarily assisting or prompting the authors to make all these enhancements.
As it happens, the exact same features necessary to support accessibility for users with disabilities can also greatly improve results in AI reuse and extraction scenarios. Authoring applications that provide AI writing support but do not thereafter generate Tagged PDF have only implemented half a solution (and are impeding all AIs that consume these documents in the future)!
On the consumption side
AI systems have a massive appetite for both data and computing resources and can consume vast troves of content from many types of systems across many different formats. With an “estimated 3 trillion PDF documents on the planet”, PDFs are a very attractive source of data. Even so, AI developers often seem to lack “situational awareness” in terms of recognizing when the data fed to their AI is correct and meaningful, and whether the ingestion tools they choose are simply up to the task.
The choice of ingestion tool(s) drives the quality of results from content. Is your HTML parser understanding ARIA roles? Does your PDF parser process the full richness of PDF?
As a crude example, there are demonstrable live AI systems today that have “learned” mojibake and “understand” complete gibberish as a Slavic or Asian language! The root cause of such problems often lies in the use of inadequate and outdated technologies that do not support proper text and content extraction from PDF files.
Another approach seen too often is to “dumb everything down” as part of an attempt to support input from pure images of documents (TIFFs, JPEGs, etc). In this case, otherwise rich documents (including, but not limited to PDF) are simply rendered to pixels and then OCR-ed to achieve a form of “consistency” for consumption by the AI engine. Not only is this computationally expensive, but all existing rich semantics and metadata is ignored and replaced by a guess from the OCR process.
Yet another very serious issue making bad headlines for AI systems arises from their unconsented consumption of Personally Identifiable Information (PII) and copyrighted content. This article doesn’t address the ethical or legal issues except to point out that PDF (and other formats) support various means to identify and protect content including encryption, digital signatures, and well-defined metadata (such as Dublin Core and C2PA).
Conclusion
Today, most AIs are fed with lousy and/or dumbed-down data that contributes to bias and untrustworthy results- and this is all before the problem of malice.
To become truly reliable, AIs need schemes for preserving rich semantics and data when they encounter it. Let the author’s AI help the author to provide this richness, and let consumer AIs leverage it when it’s provided.